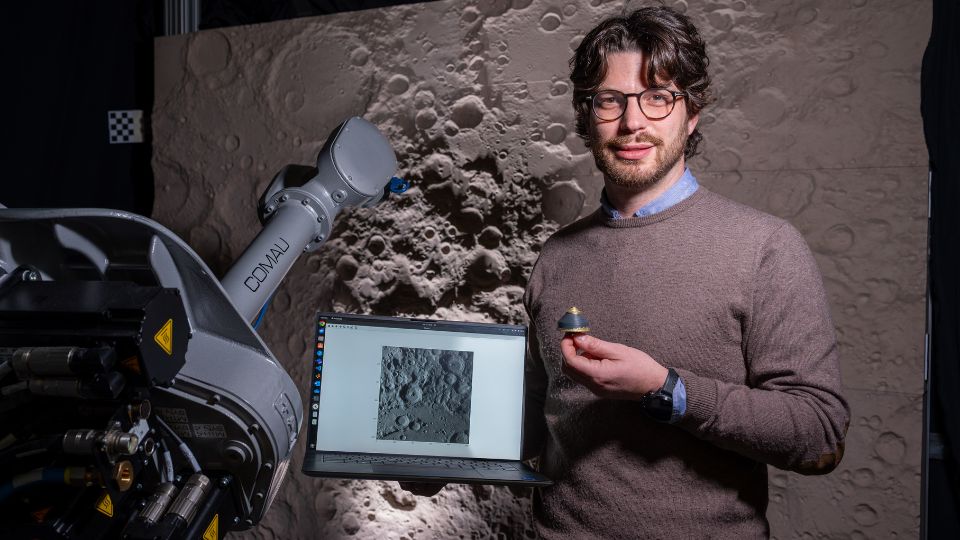
We interviewed Stefano Silvestrini, a researcher at Politecnico di Milano’s Department of Aerospace Science and Technology, who told us about his research project that was among the winners of the prestigious IDEA League Fellowship 2024-2025.
The IDEA League, comprising five leading European universities—Politecnico di Milano, TU Delft, ETH Zurich, RWTH Aachen University, and Chalmers University—fosters inter-university partnerships and networking. It enables researchers to work together to address some of the most complex scientific and technological challenges.
In this interview, Silvestrini shares his space research journey. He focuses on the challenges of autonomous guidance, navigation and control (GNC) systems for space vehicles, and offers insights from his academic and research experiences.
Can you tell us about your academic background and how you came to pursue this line of research?
I earned my Bachelor’s degree in Aerospace Engineering in Padua, where I quickly realised my strong interest in space-related topics. My academic path led me to Boston University, where I pursued a course in orbital mechanics—driven by my passion for engineering and advanced mathematics. After graduating, I continued my studies in Delft with a Master’s degree in Space Engineering. The programme included a year of theoretical coursework followed by a second year of hands-on experience through a company internship.
At that point, I realised that research was my true calling. Working in industry felt repetitive and structured, whereas I was more naturally drawn to the dynamic, exploratory nature of laboratory work. That clarity motivated me to apply for a PhD at Politecnico di Milano, where I was accepted into Professor Michele Lavagna’s Astra Laboratory.
What was your Politecnico PhD experience like?
I could pursue research in my area of interest—guidance, navigation and control (GNC) of satellites. At the time, GNC research was still emerging. We had just begun to explore the potential of artificial intelligence and were working largely through trial and error, testing what could be achieved with classical algorithms and how learning techniques might improve them. It was incredibly motivating to be involved during such a pivotal period of innovation.
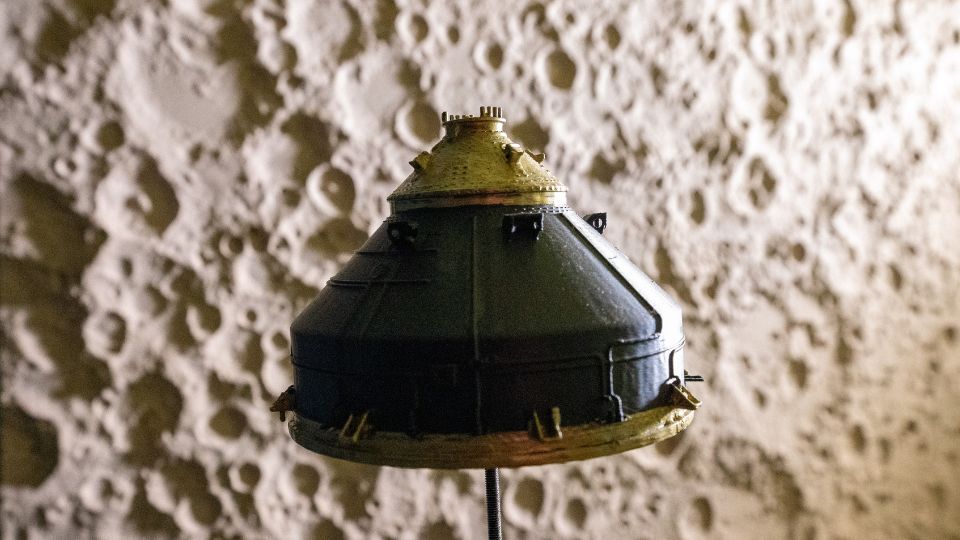
You were selected for the IDEA League Fellowship 2024–2025. Why did you return to Delft, and what was that experience like?
I returned to Delft because it was the most aligned with my field among the IDEA League universities. During my three-month research stay at TU Delft, I focused on advanced deep learning techniques for spacecraft GNC, especially for coordinated flight and proximity operations.
I worked at the Orbital Mechanics Laboratory – an excellent research environment equipped with cutting-edge robotic systems, which significantly supported my work. I could develop and test vision-based navigation algorithms in a hands-on setting.
The most rewarding part of the experience was the network I built. Connecting with researchers from different disciplines sparked a range of approaches to similar problems. That diversity of perspective led to original and effective solutions. I came away feeling deeply fulfilled—professionally and personally enriched by the experience.
Let’s talk about your project. What is the main challenge in achieving autonomous GNC for spacecraft?
The biggest challenge is enabling spacecraft to operate autonomously, as communication with Earth is not always possible. They must be capable of making real-time decisions without external input. There is significant signal latency to consider, meaning commands from Earth can’t be executed instantly. In complex missions—like approaching an asteroid, docking with another satellite, or landing in remote locations such as the Moon’s far side—autonomy is essential. In these scenarios, waiting for instructions from Earth is simply not an option.
Recently, at the Astra Laboratory, I helped launch six shoebox-sized satellites. Despite their low construction cost, operating them requires substantial time and human resources. A large team is needed to monitor them and send instructions for each action. This creates a significant imbalance between the minimal hardware cost and the sustained operational effort from Earth.
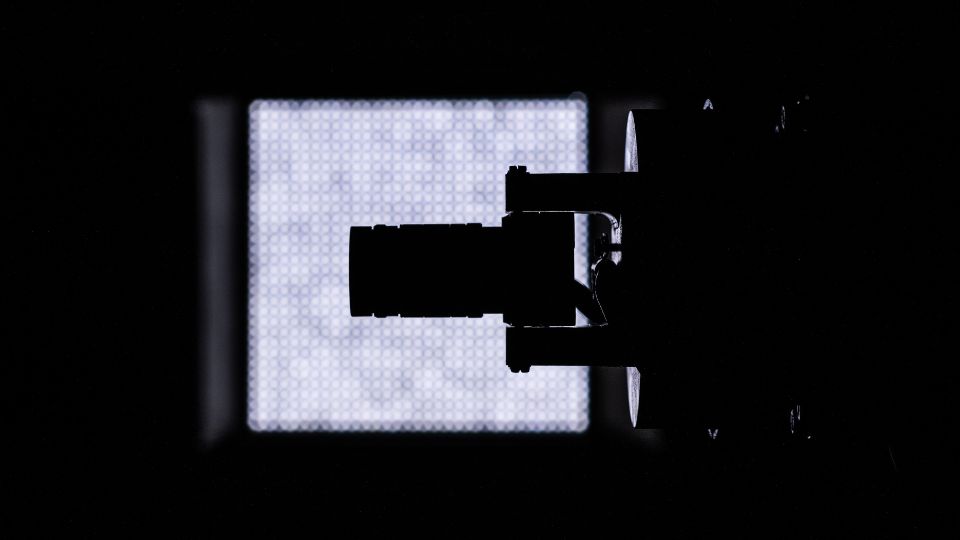
Are there emerging trends in this area of research?
Absolutely. A major shift underway is moving away from massive, billion-euro missions towards deploying constellations of smaller, cooperative satellites that can collectively achieve the same objectives.
This new approach offers several advantages: smaller, standardised platforms are simpler to develop and manage; mission resilience increases; if one satellite fails, others can be reconfigured or replaced without compromising the mission.
However, coordinating multiple satellites presents complex engineering challenges. These satellites must autonomously exchange data, determine their positions relative to each other, and coordinate their actions.
Although coordinated flight is in its early stages, ongoing developments suggest that it could significantly reduce mission costs. Once satellites are capable of autonomous operation, ground teams can focus on monitoring and data collection, rather than issuing detailed operational commands.
How can deep learning techniques improve spacecraft autonomy?
Deep learning enables systems to respond to unexpected scenarios beyond their original programming, making navigation and control more flexible and robust. These algorithms adapt to their environment in real time, which significantly increases a spacecraft’s ability to operate reliably in dynamic conditions.
My focus lies in how control laws are informed by environmental knowledge. Traditionally, this knowledge is based on mathematical models that must be simplified for satellites with limited computational power.
However, “online” learning techniques allow a satellite to refine its understanding of its surroundings during flight. This means we no longer need to rely solely on analytical models that attempt to capture every perturbation or unknown variable. The satellite learns and adjusts autonomously, becoming more precise and responsive as it gathers more data.
The more it understands its environment, the better its decisions and control actions. In essence, it adapts to the conditions it encounters in real time.
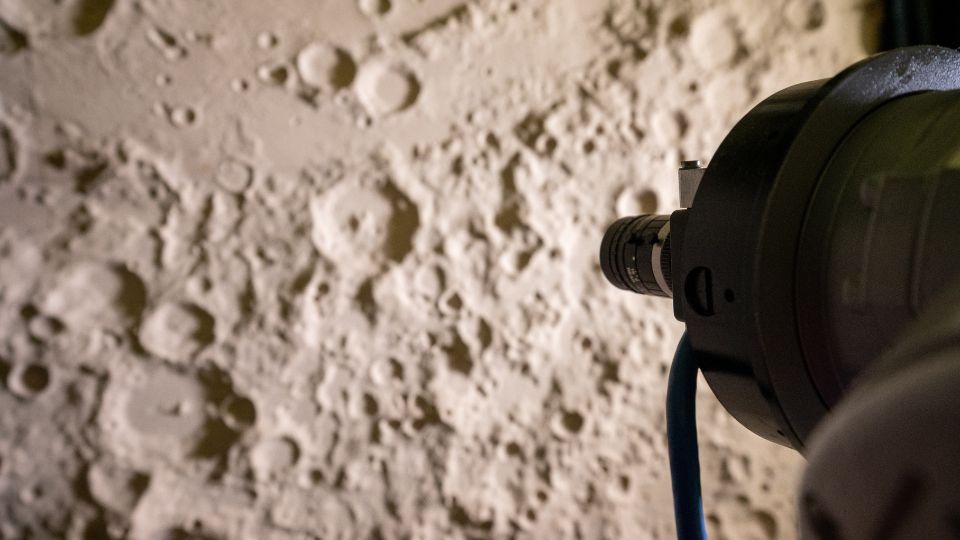
Biological intelligence—especially the human brain—has long inspired AI in navigation and control systems. Which aspects of the brain have influenced modern technologies?
One area of research argues that AI should mimic how the brain functions. This approach is rooted in the idea that by understanding how biological neurons process information, we can create more effective artificial models. Artificial neurons are the core components of neural networks, which power many AI systems today.
However, there is a debate about how these artificial neurons should be designed. Some researchers argue that they should replicate the internal dynamics of biological neurons, including the way they generate electrical impulses known as “spikes.” While this biologically detailed modelling is intellectually appealing, it has practical difficulties. The more complex the mathematical representation becomes, the less effective it proves in real-world applications. Additional complexity does not always lead to better results in AI practical implementation.
An interesting alternative is neuromorphic architectures. These models aim for a middle ground—they don’t replicate every detail of biological neuron behaviour, but they go beyond simple mathematical abstractions. The goal is to preserve the essential features of neuronal interactions in a simplified and computationally practical form.
Neuromorphic models have shown significant potential. Although they lag classical neural networks in terms of overall performance, they require far less energy and computing power. This efficiency makes them well-suited for sustainable, scalable AI.
In short, drawing inspiration from biology remains a compelling and complex area within AI development. While artificial neural networks aim to replicate brain-like connectivity, neuromorphic models offer a more efficient yet functionally relevant alternative. This line of research is paving the way for future AI systems that are not only powerful but energy efficient.
How do you integrate physics-based models into predictive control, and why are they important in unforeseen scenarios?
There are two main approaches to predictive control. The first approach relies on data: the system processes large volumes of information to determine how to respond to different scenarios. However, this method ignores the fundamental physical laws that govern system behaviour, such as gravity.
The second approach integrates physical models. In this case, the system uses data and established physical laws, such as Newton’s laws of motion, to inform its predictions. As a result, when the system makes predictions, it considers the data and the underlying physical laws.
Still, not every factor can be perfectly modelled. To handle uncertainty, the system learns to enhance these physical models with additional data, improving its ability to predict and adapt—even when faced with incomplete or unpredictable information. In this way, the system remains grounded in physics but gains flexibility to respond to real-world complexity.
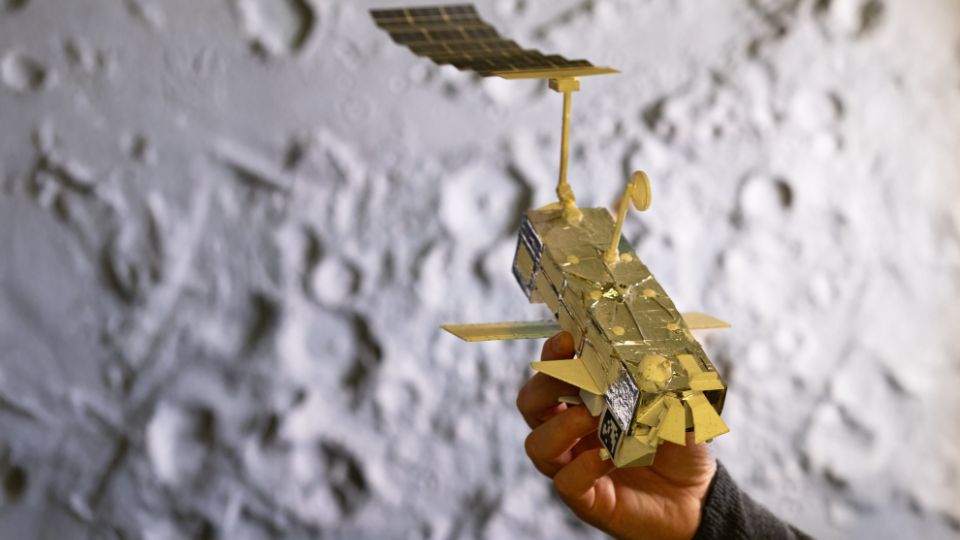
How do you simulate space conditions in the lab, and what challenges do you face in testing algorithms designed for orbit?
In the lab, we aim to recreate the conditions that instruments and robots will encounter in space. However, it’s important to acknowledge that simulations are never exact. We can control lighting and reduce friction using low-friction platforms, but we cannot eliminate Earth’s gravity affecting device movement. This means that a robot fitted with motors designed for zero-gravity conditions would behave differently in the lab than it would in orbit.
To overcome these limitations, we develop models that approximate real space conditions as closely as possible. We test algorithms under different scenarios, using imagery captured by space probes—for instance, of the Moon—to evaluate how algorithms would perform in mission environments. Although we cannot guarantee certain results, we focus on identifying performance indicators that give us confidence the algorithms will function reliably in orbit. This process of modelling, testing and refining is central to our work—and a particularly rewarding aspect of engineering research.
What stage is your project at?
We’re in a highly active phase of research and development, especially in the areas of satellite autonomy and lunar exploration. There is growing interest from space agencies and private industry in commercial applications for autonomous spacecraft. The long-term goal is to develop vehicles capable of landing autonomously on the Moon and operating independently in orbit—whether to repair damaged satellites or refuel them to extend their operational life. However, reaching full spacecraft autonomy will require several more years of research and development.
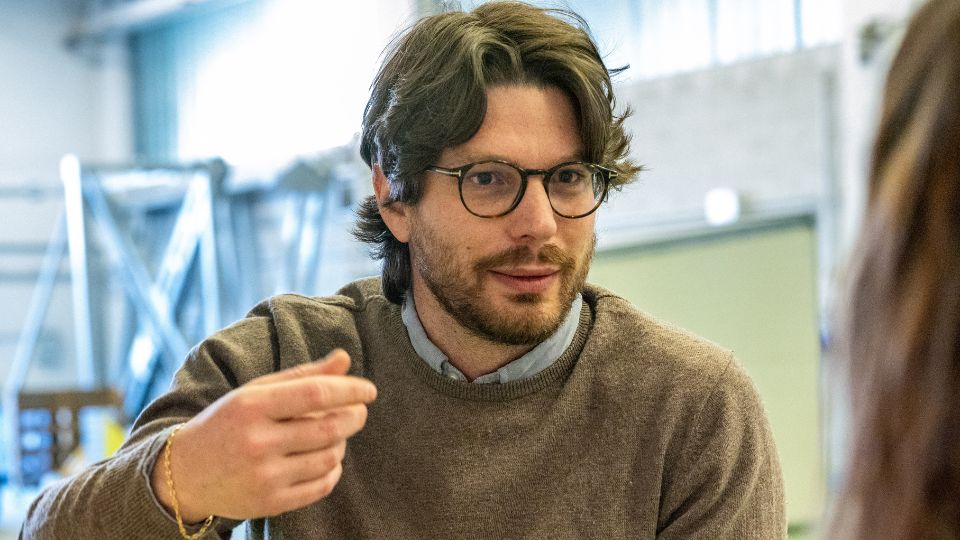
What are the main obstacles to achieving complete spacecraft autonomy?
One of the key challenges is building trust in the artificial intelligence algorithms responsible for autonomous control. Many users and professionals remain cautious about relying on algorithms that autonomously learn from data. To address this, researchers are working on explainable AI—methods that clarify how and why algorithms make specific decisions. The aim is to increase transparency and provide a level of certification that allows users to trust the actions taken by autonomous systems. However, there is an ongoing debate. Some argue that limiting algorithms to make them comprehensible could reduce their potential. As a result, research continues to focus on finding the right balance between advanced autonomy and human interpretability—crucial for the safe deployment of autonomous spacecraft.
Are there real-world applications for this research outside of space exploration?
Absolutely. While much of our work targets space missions, many of the technologies and methodologies developed at the university have broader applications.
Robotics and autonomous guidance are developing rapidly. Unlike space environments—where testing is expensive and logistically complex—Earth-based trials are much more accessible. It’s far simpler to install a camera on a car and test it in traffic than to deploy a rover on another planet.
Although the challenges differ, techniques originally designed for space can often be adapted to terrestrial contexts, contributing to improvements in everyday technologies.
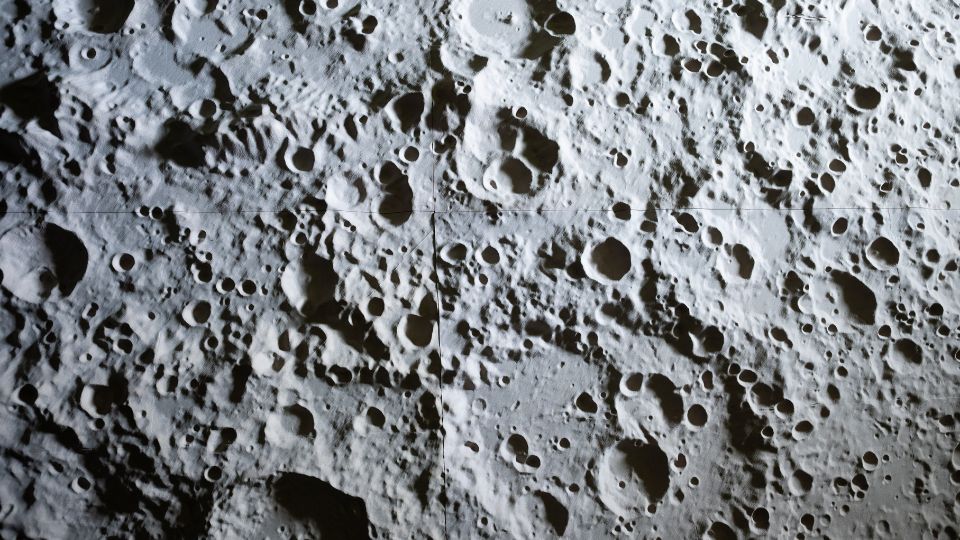
What excites you most about your research?
I really enjoy my work, especially anything to do with space. But my interests go beyond that. I’m particularly drawn to the broader applications of my research. I explore how algorithms can be adapted for other domains.
One of my ambitions is to improve navigation systems. Traditionally, we treat guidance, navigation and control as separate modules. However, on closer examination, it becomes clear that these distinctions are not always so sharply defined, particularly in computing. Our brains process movement and orientation holistically—even though certain brain areas are specialised, the system works as a unified whole.
In my future, I would like to further explore how we understand our position and decide where we want to go. We don’t consciously calculate distances or angles while walking. We navigate smoothly and efficiently without thinking about it. This “unconscious navigation” is something we have yet to replicate in machines. It is an ambitious goal, but I would love to explore how integrating neuroscience and computer science could help us get closer to human-like perception and decision-making.
What motivates me most is the transition from theory to application. Each time an algorithm performs as expected, it’s incredibly rewarding. The idea that I’m contributing to a future of autonomous spacecraft is a source of inspiration.